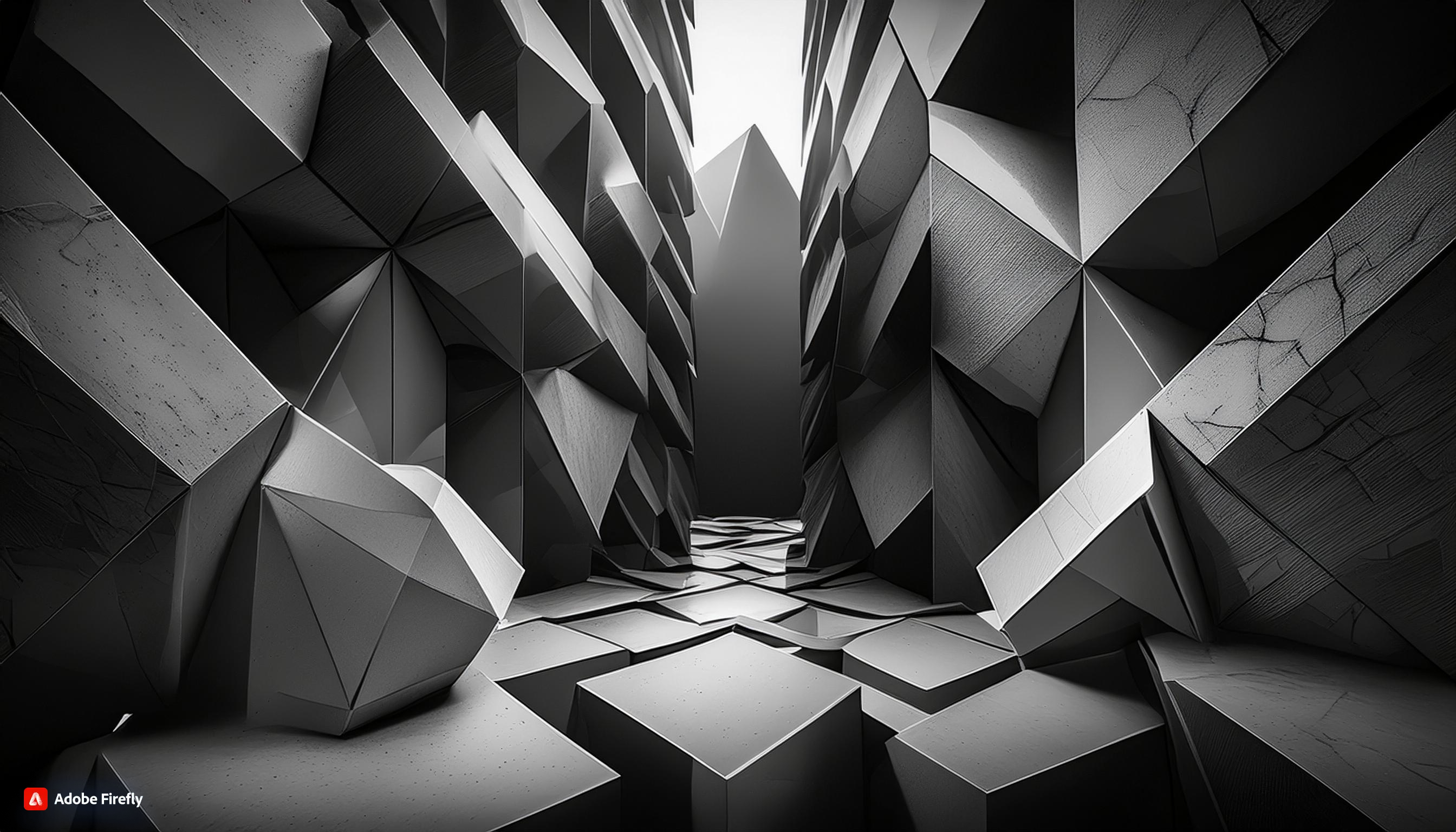
Late last year I finished the paper I referenced in my my previous post. I'm going to post the core argument in it, skipping out a few contextual sections that primarily deal with the underlying technology of AI and its history.
This field is growing and changing so fast, I feel like these posts might be laughable in a few years, but I still feel it is an important discussion topic while we are in the throws of the popularization of these tools.
The Flaws in "AI"
The first task is to explore several of the core flaws and concerns around these tools. These are presented in no specific order, and to a large degree are all interrelated with each other and the concept of training. These flaws include, and are not limited to, issues of copyright, ethics, and cultural bias.
Of all these topics the easiest entry point to the discussion is likely copyright. As previously stated, these tools rely on terabytes of data that have been collected from the internet, and in most systems this content has been collected without the consent (Growcoot, 2023), compensation, or even awareness of most individuals whose data has been collected. This has put the legal status of these training sets in a precarious position (Brittain, 2023). Multiple groups have come out against the use of their data in AI training sets. Ranging from artists and chefs to open-source software developers and actors (Dalton, 2023) various groups have begun action against these efforts including litigation, strikes, and opt-out requests. (Hays, 2023)
A close cousin to the problem of copyrights on the data used in training sets is the compensation of the creators of that data. Companies like Reddit have made deals with other AI companies to license the content their users post on their platforms but are not making strides to compensate the actual creators of that content (Isaac, 2023). There are people proposing compensating individual artists and creators, but due to the black-box nature of neural networks deciding what images are used to generate another image is virtually impossible. This leaves the tasks of attribution, compensation, and decision auditing as some of the largest flaws in AI systems. This inscrutability makes it so that a researcher cannot ask or investigate why an AI tool outputs a specific image or text.
Compensation may be possible if you ask a LLM to “write a joke in the style of Lenny Bruce” or “create a painting of a cow in the style of Banksy”, but that is only one aspect of the creation of a piece of content. How many thousands of images of “cows” or descriptions of jokes make up the rest of the “decisions” going on within the neural networks? The sheer scale of data used makes attribution and compensation a very difficult problem. (Noveck & O’Brien, 2023)
Coupled closely to the problem of compensation and copyright is the legal risks around the use of these tools. There is still an open question in the eyes of many legal systems as to if there is liability in the use of these tools due to the lack of attribution and licensing of the data used to create their model. There are several open lawsuits from creators against AI companies to seek either compensation or the removal of their data from training sets. The one question that was answered recently by the US legal system was the ownership of the output of an AI model, to the eyes of the court no one can copyright the output of a model. (Brittain, 2023)
The ethics of computer systems is a huge and complex topic of discussion that spans from everything from piracy, the dark web, free speech, and surveillance. Each and every ethical dilemma that might be found on the internet can be found within the space of AI tools. Some tools have been reported to be using human labor to fake AI output (Cox, 2023), some sites offer the ability to train models to create non-consensual images (Maiberg, 2023d) or voice recordings (David, 2023) of other people, and some AI tools are being created without even a basic moral code, allowing them to encourage self-harm, or terroristic intentions. (Maiberg, 2023c)
While not designed to imply AI is the end of humanity, the above paragraph is intentionally inflammatory, and hopefully gives the reader pause. There are a group of people within the AI community who are advocating for a pause as well. The “slow AI movement” is calling for taking a more thoughtful measured approach to developing these systems, potentially even including a full pause in AI development. Detractors of this movement call it a restriction of vital new technology or even an anti competitive bid to allow the largest players in the space time to lobby for legislation which could keep them at the front of the field.
A final topic that should not be overlooked with regards to flaws in AI is bias. At this point it is a given that broadly speaking, AI tools are trained on the data available on the internet, and while the internet is an open democratic platform, it is not an unbiased one. Access to the internet is heavily focused on the developed, English speaking world, and as a result underdeveloped or developing populations and other cultures are underrepresented. This lack of representation can translate directly to the output of image generation models or LLMs. LLMs are woefully lacking in their ability to interpret and create content in language outside of the top handful (Deck & Deck, 2023) for example.
Lack of representation is just one side of biases that can be found in these systems, another is stereotyping. Given their nature of "averaging” data to create a best guess of what something is, these tools are incredibly prone to stereotyping (Turk & Turk, 2023), and making assumptions. Requests to midjourney to generate a “Mexican person” almost all included a man in a sombrero, or people generated tend to be male, or “doctors in Africa” were largely white (Drahl, 2023). This is troubling in a few aspects as gender was assumed male, and a vast culture was reduced to a hat. These examples, while potentially trivial in the context of generating an image for marketing purposes, could have a very different conation when used in a tool to generate police sketches for criminal suspects (Maiberg, 2023b). While the bias of these tools reflects their training data, and to a degree that can be compensated for, their inherent systems require them to average vast quantities of information, which makes this process very difficult to surmount.
Additional concerns around AI include but are not limited to the cost and environmental impact of training models (Calma, 2023), the ingestion of personal identifiable information, and the replacement of jobs via AI tools. Each of these are valid concerns which warrant further discussion but are being left out for the moment.
In summary, AI tools face significant challenges, including copyright issues related to unauthorized data use and disputes over compensation for data creators. Legal uncertainties persist regarding liability and ownership of AI-generated outputs. Ethical concerns involve misuse for non-consensual content creation and the advocacy of a more thoughtful development approach. Bias in AI, stemming from underrepresentation and stereotyping, remains a pervasive issue, affecting the accuracy and fairness of outputs.